By Chris Cook, PharmD, Ph.D., US Medical Advisor and Director, US Medical Science Liaison Team at bioMérieux
Scientists and clinicians have typically used two main approaches to study the processes that drive antimicrobial resistance—clinical studies focused on identifying resistance genes and the epidemiology of resistance, and experimental studies focused on understanding how antimicrobial resistance changes in response to selective pressures. However, experimental studies performed in research labs “have not placed enough emphasis on understanding the evolution of successful strains that have driven the rise of resistance in the clinic,” according to a recent article published in Science.1
The authors of the Science article note that while there are many studies that have investigated the fitness of laboratory-evolved resistant organisms, our understanding of the fitness of “naturally” evolved clinical pathogens is still limited. There are key differences between laboratory and clinical settings that affect the ways that antibiotic resistance evolves, including the microbial communities that organisms live in,2 their lifestyles as part of a biofilm or as independent microbes,3 and environmental selective pressures.4
Bacterial Communities & Horizontal Gene Transfer
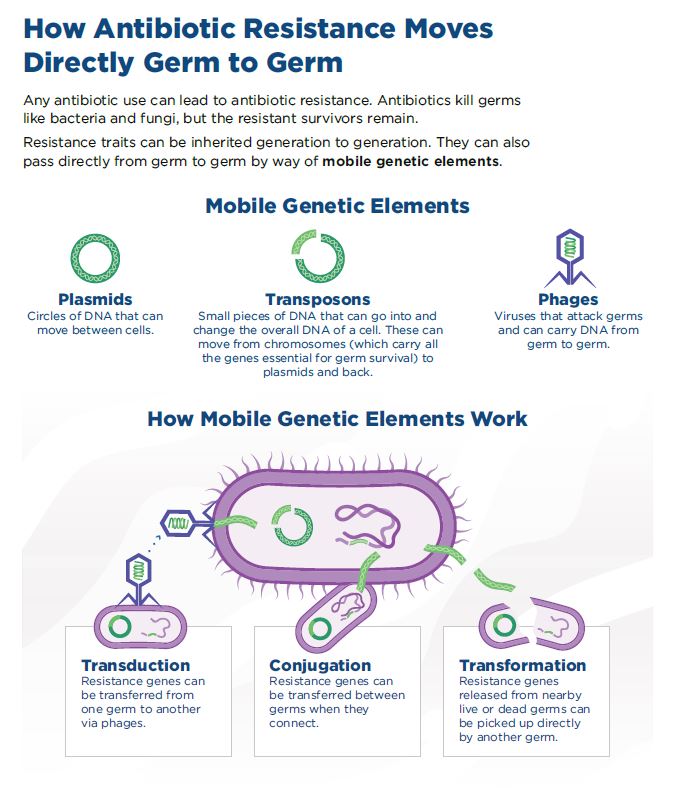
In the human body, bacteria don’t live in isolation; instead, they live in communities that consist of many types of bacteria, a critical difference from a typical research laboratory environment. Most bacteria that live in the human body are harmless, and some are necessary for maintaining a healthy, functioning body. However, bacterial communities can provide a rich source of resistance genes, located in mobile genetic elements that can be transmitted from one bacterium to another through the process of horizontal gene transfer (HGT).1
While the basic mechanisms of HGT were discovered over 50 years ago, the main challenge today is to better understand the rate at which bacteria acquire resistance genes through HGT, alongside improving our understanding of the conduits for gene transfer. The authors of the Science article suggest that, “It is possible that studying patterns of distribution of ABR [Antibiotic Resistance] genes in microbiomes combined with experiments that track the acquisition of ABR genes in complex communities could help to start solving this puzzle.”
Bacterial Lifestyle
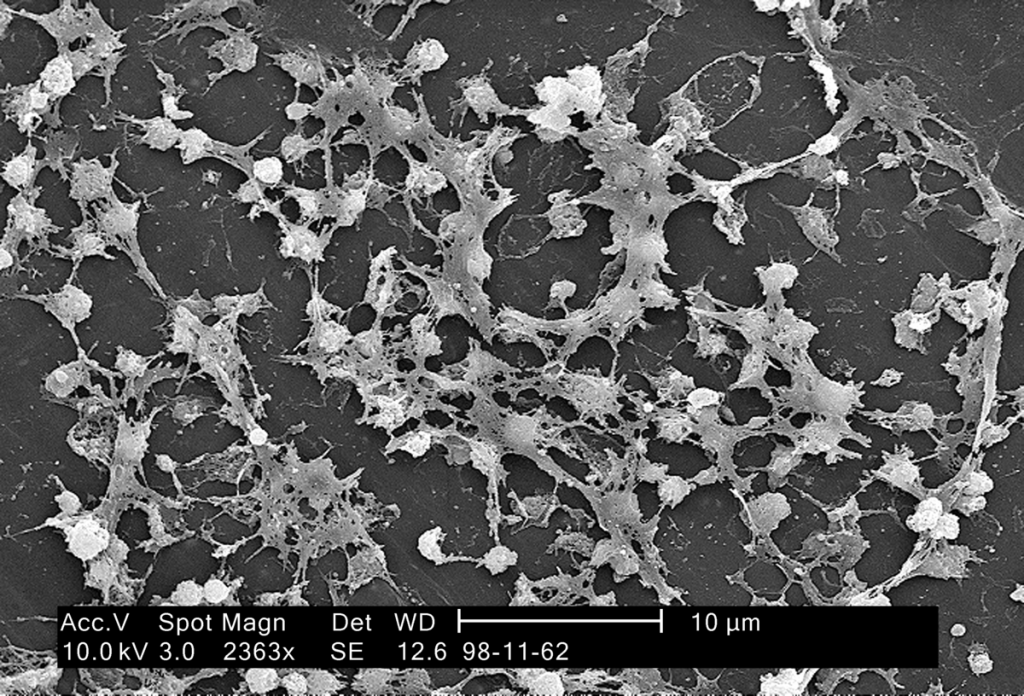
Another difference between typical laboratory and clinical environments is bacterial lifestyle. A study published in October by researchers at the University of Pittsburgh, titled, “Evolutionary pathways to antibiotic resistance are dependent upon environmental structure and bacterial lifestyle,” suggests that how bacteria live, whether as independent cells or as part of a communal biofilm, determines how they evolve antibiotic resistance.3
The collective of microorganisms that form a biofilm produce substances to bind themselves to a surface and to other microbes in the community, which ultimately results in alteration of microbial growth rate and gene transcription.5 According to a coauthor of the Pittsburgh study, Michelle Scribner, “Biofilms are a more clinically relevant lifestyle. They’re thought to be the primary mode of growth for bacteria living in the body.”
Evolutionary Selective Pressures
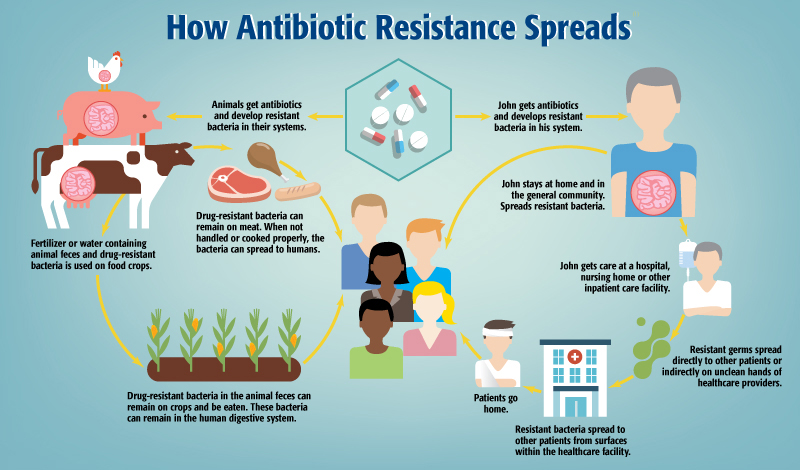
Clinicians must also contend with a range of external environmental factors that combine to shape the emergence of antimicrobial resistance. These factors include the use of antibiotics within their own facilities, but also patients who use antibiotics unnecessarily and without medical supervision, which is especially common in countries where antibiotics are available without prescriptions.
Antibiotic pollution in the environment has also been shown to be high enough to exert selective pressures leading to resistance.4 The improper use of antibiotics in agricultural settings can contribute to environmental antibiotic pollution,6 in addition to driving resistant infections in populations of food animals. While guidelines from agencies like the FDA are helpful,7 unnecessary antibiotic use is still prevalent in the United States and elsewhere.
Certain regions are more prone to the spread of certain diseases,8 and more generally, infectious diseases spread faster when there are problems with community sanitation. A further complication is that some populations are more vulnerable because of age or immunosuppression, which are both common characteristics of many hospitalized patients.
The Power of Data at Scale
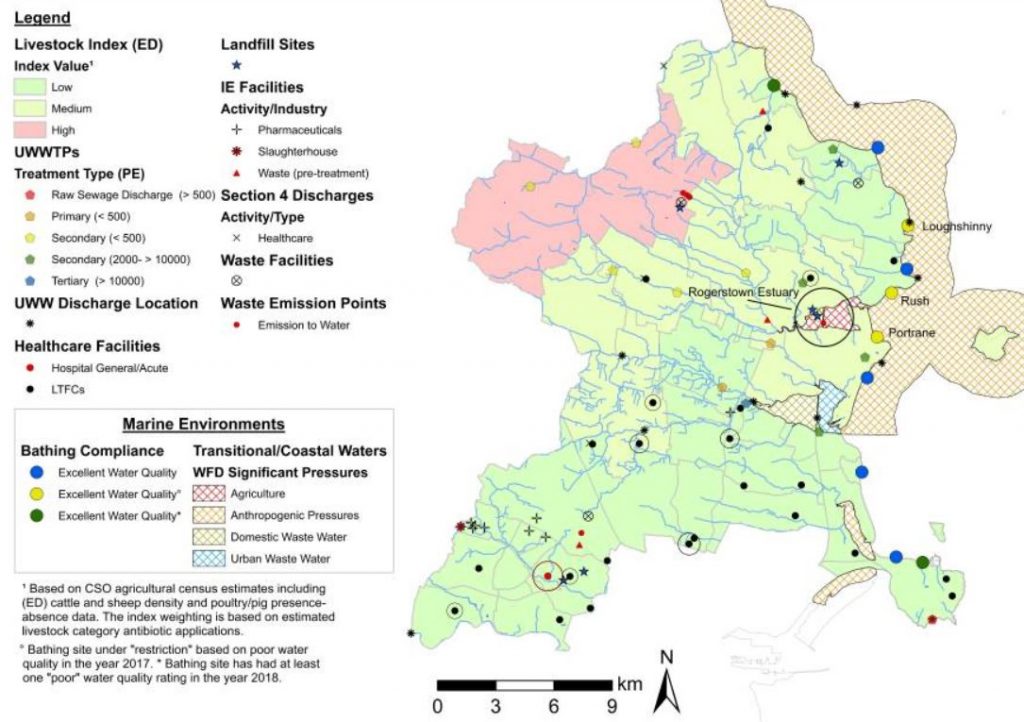
Although the sheer complexity and volume of factors that contribute to the emergence and spread of resistant infections are daunting, new tools and approaches may help us combat the threat.
Big data is one powerful resource emerging in healthcare.9 With unprecedented computing power and the ongoing digitization of medicine, it is now possible to analyze large quantities of data with speed and accuracy. Programs that leverage regional, national, and global data for improving public health are already in use through organizations like the Centers for Disease Control and Prevention and the World Health Organization.10, 11
However, individual hospitals often do not have the ability to collect data in the same way, especially in low-resource settings.12 Even large healthcare networks, who have troves of data from advanced diagnostic systems, electronic health records, laboratories, and pharmacies, may struggle to synthesize that data efficiently enough to inform clinical decision-making where it matters most: at the point of care.13
Data from electronic health records, including patient information, patient’s medical history, allergies, test results, and treatment plan can provide insights that impact individual patient outcomes, hospital operating costs, and the power to track and predict infectious disease outbreaks.14 From a research perspective, such in-depth surveillance may also offer insights into some of the evolutionary drivers of resistance, which in turn may inform a hospital’s infection control practices.15
The ability to monitor and benchmark resistant organisms, resistance genes, and other infection control dynamics can show how resistance is evolving in real time within a healthcare facility or healthcare network.16 Paired with national and global infection surveillance systems and whole genome sequencing data, advancements in data analysis at the hospital level may provide the edge that clinicians need to stay ahead of antimicrobial resistance and save patients’ lives.17
References:
- Maclean, R. Craig, and Alvaro San Millan. “The Evolution of Antibiotic Resistance.” Science, vol. 365, no. 6458, 12 Sept. 2019, pp. 1082–1083., doi:10.1126/science.aax3879.
- Alseth, Ellinor O., et al. “Bacterial Biodiversity Drives the Evolution of CRISPR-Based Phage Resistance.” Nature, vol. 574, no. 7779, 2019, pp. 549–552., doi:10.1038/s41586-019-1662-9.
- Santos-Lopez, Alfonso, et al. “Evolutionary Pathways to Antibiotic Resistance Are Dependent upon Environmental Structure and Bacterial Lifestyle.” ELife, vol. 8, 2019, doi:10.7554/elife.47612.
- Tello, Alfredo, et al. “Selective Pressure of Antibiotic Pollution on Bacteria of Importance to Public Health.” Environmental Health Perspectives, vol. 120, no. 8, 2012, pp. 1100–1106., doi:10.1289/ehp.1104650.
- Donlan, Rodney M. “Biofilm Formation: A Clinically Relevant Microbiological Process.” Clinical Infectious Diseases, vol. 33, no. 8, 2001, pp. 1387–1392., doi:10.1086/322972.
- McKenna, Maryn. “Antibiotics Set to Flood Florida’s Troubled Orange Orchards.” Nature News, Nature Publishing Group, 19 Mar. 2019, www.nature.com/articles/d41586-019-00878-4.
- Medicine, Center for Veterinary. “Antimicrobial Resistance.” U.S. Food and Drug Administration, FDA, 2019, www.fda.gov/animal-veterinary/safety-health/antimicrobial-resistance.
- “WHO Report on Global Surveillance of Epidemic-Prone Infectious Diseases.” World Health Organization, World Health Organization, 24 July 2015, www.who.int/csr/resources/publications/introduction/en/index4.html.
- Eisenstein, Michael. “Infection Forecasts Powered by Big Data.” Nature News, Nature Publishing Group, 7 Mar. 2018, www.nature.com/articles/d41586-018-02473-5.
- “NHSN.” Centers for Disease Control and Prevention, Centers for Disease Control and Prevention, 5 Apr. 2017, www.cdc.gov/nhsn/index.html.
- “GLASS | Global Antimicrobial Resistance Surveillance System (GLASS).” World Health Organization, World Health Organization, 19 Oct. 2018, www.who.int/glass/en/.
- Ashley, Elizabeth A, et al. “Harnessing Alternative Sources of Antimicrobial Resistance Data to Support Surveillance in Low-Resource Settings.” Journal of Antimicrobial Chemotherapy, vol. 74, no. 3, 2018, pp. 541–546., doi:10.1093/jac/dky487.
- Reisman M. (2017). EHRs: The Challenge of Making Electronic Data Usable and Interoperable. P & T : a peer-reviewed journal for formulary management, 42(9), 572–575.
- Kruse, C.S., Stein, A., Thomas, H. et al. J Med Syst (2018) 42: 214. https://doi.org/10.1007/s10916-018-1075-6
- Institute of Medicine (US) Forum on Emerging Infections; Harrison PF, Lederberg J, editors. Antimicrobial Resistance: Issues and Options: Workshop Report. Washington (DC): National Academies Press (US); 1998. Workshop Report. Available from: https://www.ncbi.nlm.nih.gov/books/NBK100888/
- Conlan, Sean, et al. “Plasmid Dynamics in KPC-Positive Klebsiella Pneumoniae during Long-Term Patient Colonization.” MBio, vol. 7, no. 3, 2016, doi:10.1128/mbio.00742-16.
- Monk, Jonathan M. “Predicting Antimicrobial Resistance and Associated Genomic Features from Whole-Genome Sequencing.” Journal of Clinical Microbiology, vol. 57, no. 2, 2018, doi:10.1128/jcm.01610-18.
Opinions expressed in this article are not necessarily those of bioMeriéux, Inc.